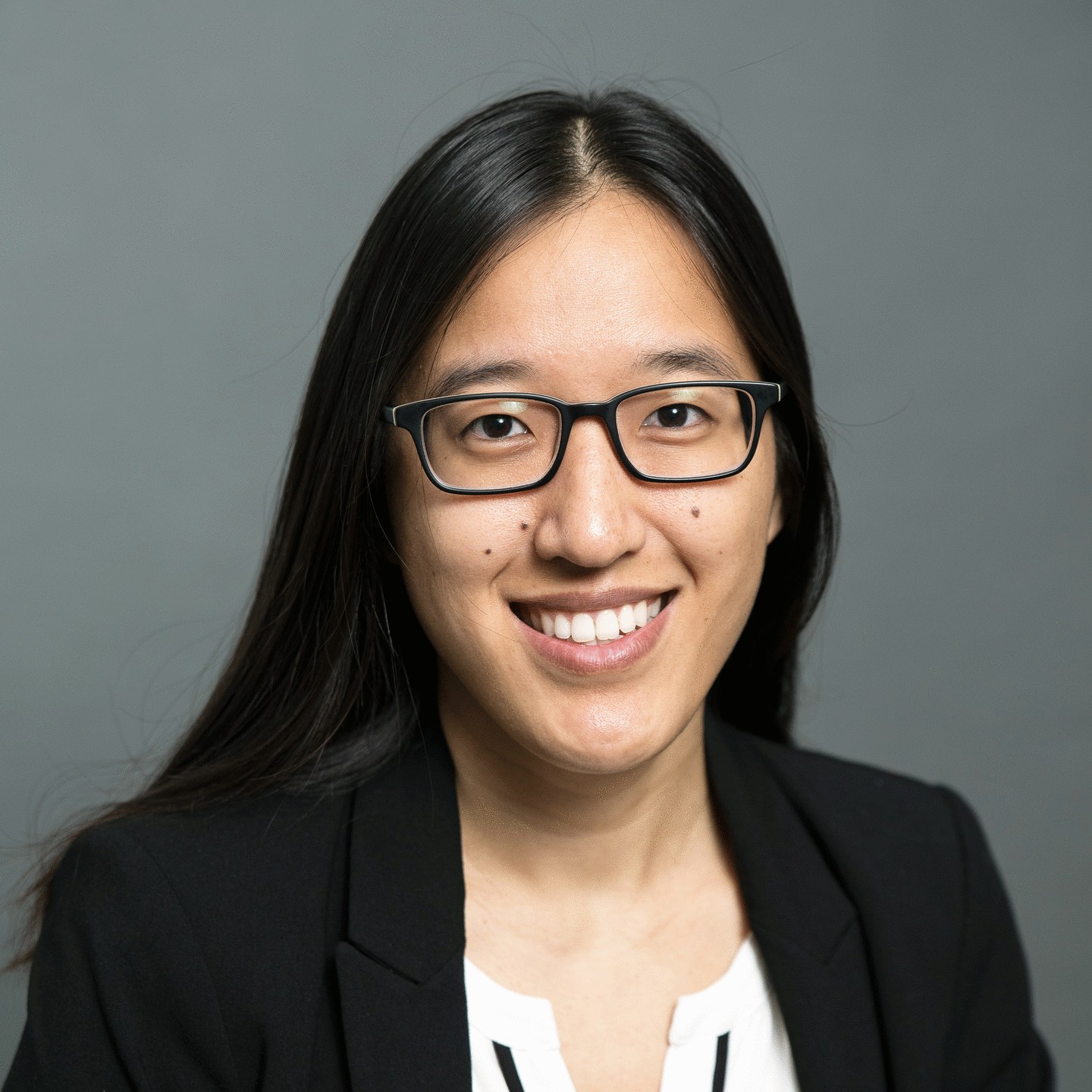
Causal Inference in Marketing using Synthetic Control (and related) Methods
Marketing researchers are interested in understanding causal relationships in a variety of contexts. While randomized experiments are the gold standard for establishing causality, they are often not feasible. In such cases, marketing researchers can leverage quasi-experimental data and methods to still establish causality. In the last two decades, there has been a renaissance in the development and improvement of quasi-experimental methods for understanding causal relationships. In this presentation, I will present an overview of recent developments in the quasi-experimental methods and their marketing applications. Specifically, I will focus on my contributions to the literature including developing the inference for popular methods such as the synthetic control method and the factor model methods (aka the generalized synthetic control method) and proposing new methods such as the Augmented Difference-in-Differences method and Forward Difference-in-Differences method. I will also discuss how to formally check identifying assumptions and how to choose the appropriate quasi-experimental method based on the data characteristics of the research problem.
Presenters
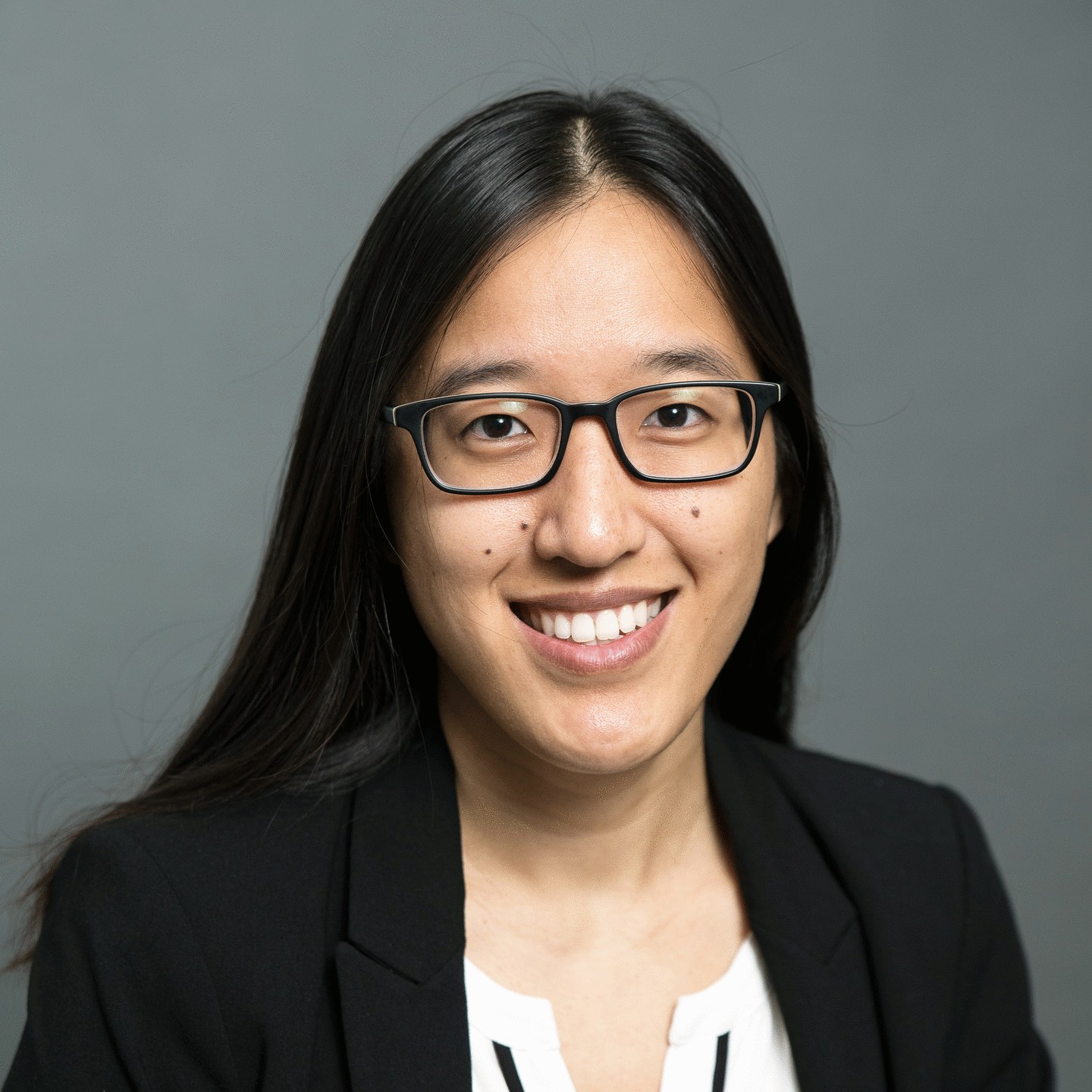